Having an accurate estimate of wind resource is a key first step in understanding wind farm performance. The problem that haunts most wind energy analysts is that on a fundamental level, measurements of wind resource with use of conventional anemometry are challenging and plagued with inaccuracies, signal drift, recalibrations, and data logging issues. Whilst efforts are being made to improve this measurement capability (for example nacelle mounted LiDAR), those who monitor the performance on wind farms are left to clean up the mess in the meantime. To that end, at Bitbloom we have developed a new approach to gain confidence in local turbine wind speed measurements, which is being rolled out across all the wind farms that we monitor.
So how do we improve a signal such as a wind speed measurement and generate something more reliable. Beyond the typical noise associated with these signals, a more fundamental question, given our large volumes of data, is: Can we trust that the observed values are unbiased? Well, we can attempt to calibrate our measured signal using other sources of information. This is commonly done through various means, including reverse engineering expected wind speed using other measured values, such as power (which is typically more accurate), or through completely different datasets altogether, such as climate reanalysis data (see ERA).
Many of these approaches seem to overlook one important asset that analysts have at their disposal, and that is the (often substantial) number of other turbines on the site. These turbines are fitted with their own monitoring systems and generate similar data, giving analysts a wider view of site conditions. As wind speed conditions across a wind farm site are generally strongly correlated, the operational behaviour of neighbouring turbines can be used to infer wind conditions at a nearby position, such as that of a turbine with questionable anemometry.
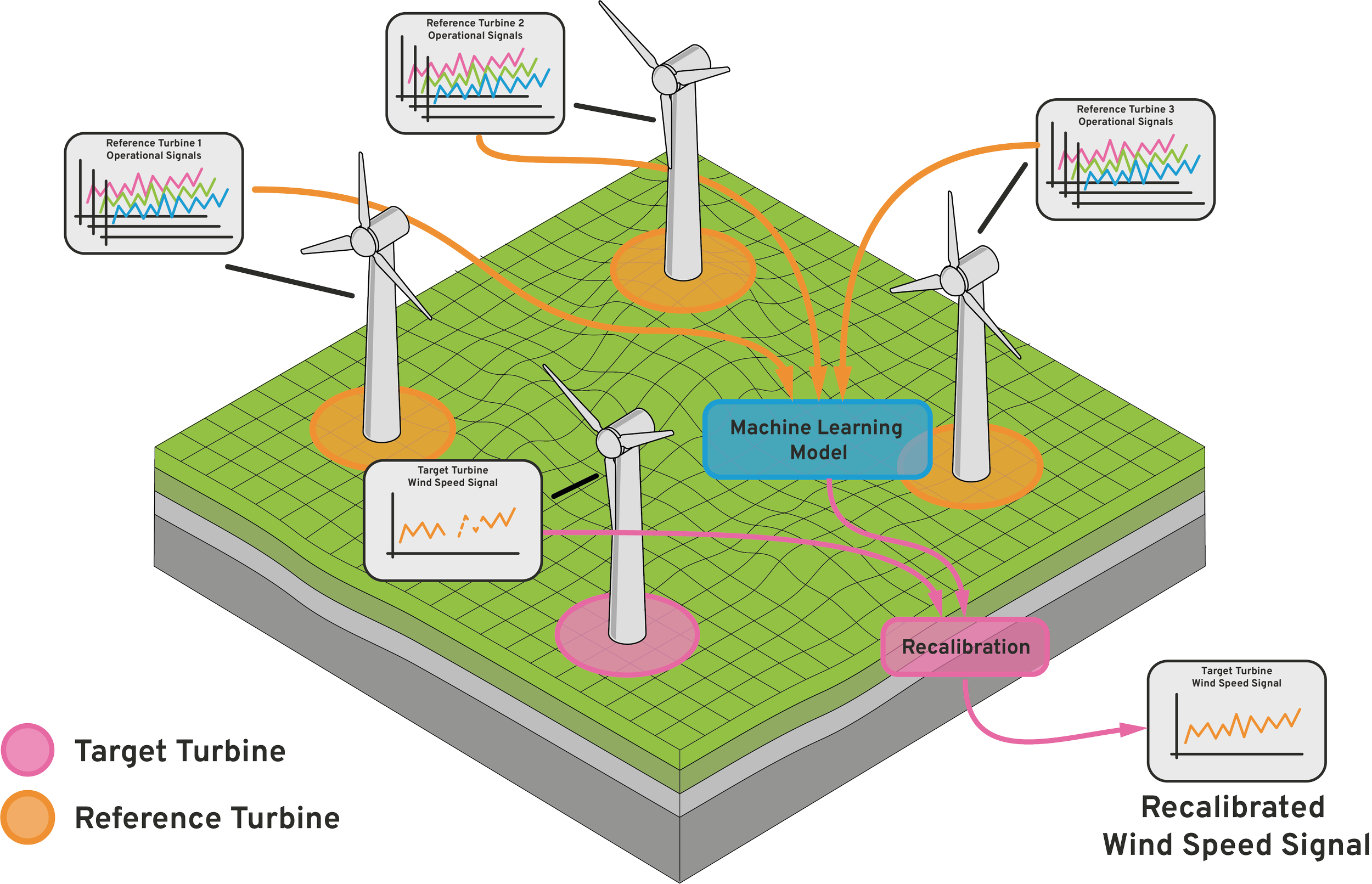
Okay, great, we’re on to something. We can leverage the operational signals from the neighbouring turbines to reconstruct our wind speed measurement, effectively using them as giant anemometers. It’s a similar (albeit less potentially catastrophic) principle to multiple instruments in aircraft, aggregating measurements in such a way enables us to have a much greater degree of confidence in the measurements we are basing our decisions on.
So, how can we quantify the relationship between the measured operational condition of one turbine, and the environmental conditions (i.e a given wind speed) at a different, target turbine? Here, machine learning steps up to the challenge in helping us build this relational model. Using the operational data from neighbouring turbines, we can train a machine learning model to predict the wind speed at a target turbine, capturing these relationships with a data-driven approach. This model can then be used in inference to generate an expected wind speed value for a given turbine which we can use to calibrate the wind speed that is observed. If the relative positions are also encoded into this training set, a model of sufficient complexity can even learn the effects of prevailing wind direction and generated wakes.
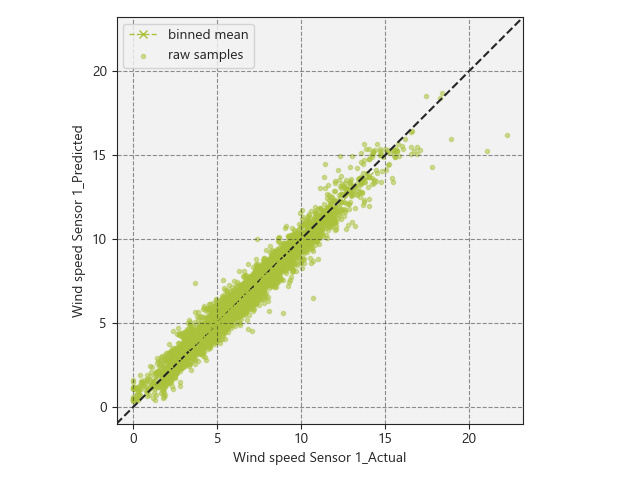
The model is trained on a dataset of sufficient length to capture the site wind regime, typically a year of data, consisting of operational signals from multiple neighbouring turbines. We use the measured wind speed, power, blade pitch angle, ambient temperature, rotor speed, and the wind direction at each neighbouring turbine as input features. Given the input features, the model is able to learn wake and terrain effects and account for episodes of curtailment and downtime and maintains performance in a wide range of environmental conditions. Similar models can be trained for all the turbines in a farm (given a sufficient number of nearby turbines) and then be used to calculate a signal of expected wind speed signal beyond the period of the training dataset, and on new incoming data.
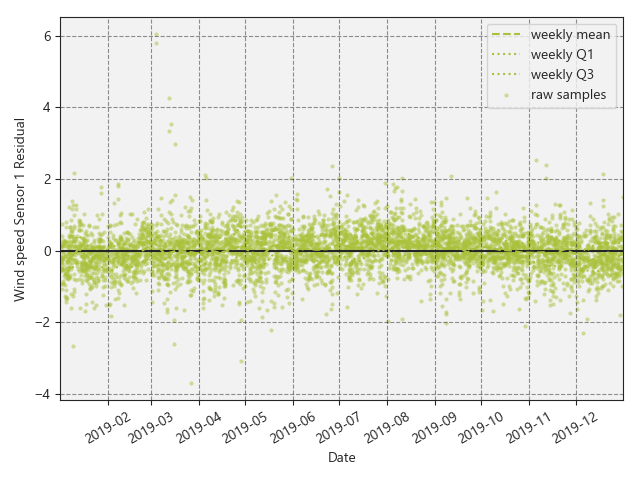
The measured wind speed is then calibrated using this expected wind speed signal based on the residual (i.e. the difference between observed and expected) between the two. This is now used to scale the observed values leaving us with a final, recalibrated measurement of our wind speed, which is then used to track performance with confidence and deriving reliable energy loss estimates.
Well, that’s that, we’ve gained confidence and accuracy in our wind speed measurements by using plentiful data at nearby turbines; allowing our customers to avoid lost yield by picking up performance issues and avoid false positives caused by erroneous wind speed measurements. This also enables us to have a greater degree of confidence in dependent turbine analyses, generating compounding value for analysts. This approach is now deployed to wind farms that Bitbloom monitors and is already providing value to our customers.