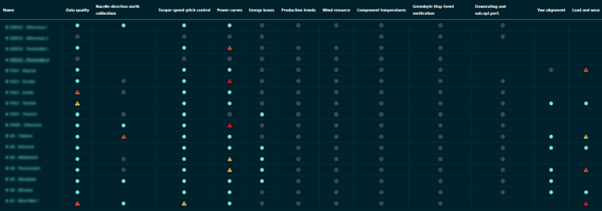
The devil in the detail
Bitbloom analysed four years of Cubico’s historical 10-minute SCADA and event log data using automation on its Sift Monitor platform. After ingesting and quality assuring the data, Bitbloom would enrich the data before applying Sift Monitor’s performance monitoring algorithms. Run on a daily basis, these analyses were made available to both Bitbloom and Cubico via the Sift Monitor dashboard, which employs a simple traffic light system to draw attention to potential issues and alert on exceptions.
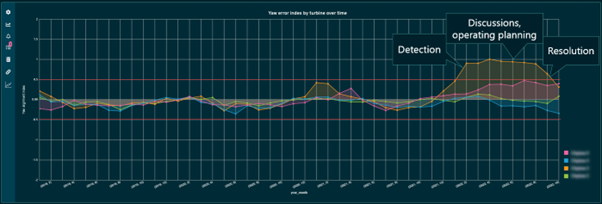
Collaboration
Bitbloom and Cubico meet regularly to discuss new issues and track progress on open items, using a task collaboration interface built into Sift Monitor. The interface is designed to both track tasks and collate relevant evidence such as analysis results.
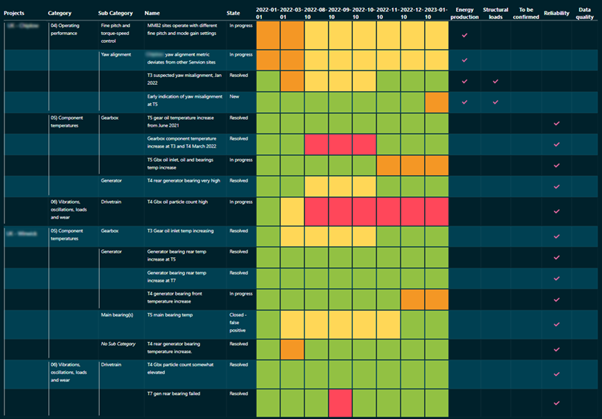
Diagnosing the issue
In one of these calls, Cubico and Bitbloom had a particular agenda item to discuss: two separate algorithms had detected an anomaly, and a third had provided a true negative which, in combination, allowed the team to diagnose a yaw misalignment issue.
- Algorithm 1: A simple power curve and efficiency tracking tool indicating potential drop in efficiency.
- Algorithm 2: A wind speed consistency tool indicating no atypical increase in resource estimate for the specific turbine and period.
- Algorithm 3: A proprietary yaw misalignment algorithm indicating potential increase in static yaw misalignment.
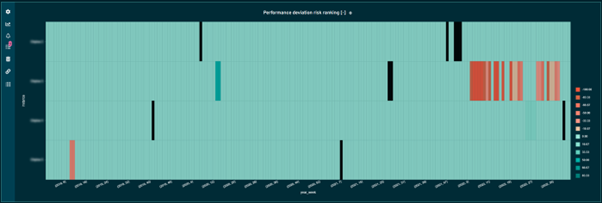
Combination of indicators
In isolation, none of these indicators would have been sufficient to diagnose and resolve the issue. However, when combined, they pointed to yaw misalignment as the likely problem. Algorithm 3 specifically pointed to this, while algorithm 1 corroborated it with a drop in efficiency that algorithm 2 confirmed was not due to increase in wind resource.
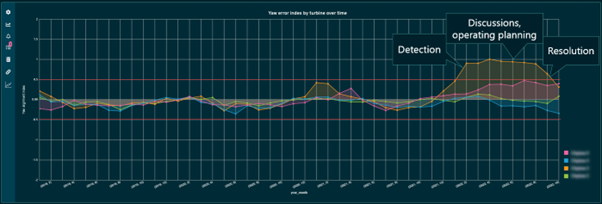
Presenting the data
For operators, it can be expensive to despatch engineers to physically investigate issues, leading to a sensible reluctance to do so without strong evidence of a suspected issue. However, Cubico was able to present the operator with the evidence compiled by Bitbloom. The strength of this evidence persuaded the operator that a physical instrumentation inspection was justified.
Probing pernicious problems
Physical inspection confirmed what the data indicated: the operator discovered static yaw misalignment in excess of 10 degrees.
With swift correction of the issue, the avoided energy loss alone was sufficient for Cubico to offset the cost of Bitbloom’s services for the entire European portfolio for an entire year. What’s more, as neither the operator nor service provider had the issue on their radar, it is feasible that it would have remained undetected for a long time, all the while accumulating fatigue damage and component wear which, though difficult to quantify, could well account for greater avoided costs in repairs and downtime than the direct energy loss.
Today, Bitbloom continues to monitor various metrics for Cubico including yaw control and alignment, power controller operation, failure prognostics through temperature deviations and oil particle monitoring. By unearthing pernicious and expensive yaw misalignment issues, Bitbloom has validated its existing-data-first approach. Cubico was able to cost-effectively sweat its existing SCADA data to uncover the large misalignments that had the greatest potential to harm operations and profitability. In future, it may choose to invest in further data collection for analysis to optimise efficiency to an even greater degree, but it can do so knowing it has already made the bigger gains at a lower cost.